Let's dive back into our conversation with ChatGPT. This week, we're focusing on security – from what IT has to be concerned about when it comes to generative AI tools being used in an organization, to how enterprises can leverage the technology to help bolster network security.
Redmond: Thanks for continuing our conversation about generative AI's role in enterprise IT
ChatGPT: You're welcome! I'm here to continue our conversation. Please feel free to ask any questions or share your thoughts on the topic, and I'll do my best to provide you with the information you're looking for.
Let's talk about security concerns. What possible risks are there to organizations by employees using generative AI technology on their network?
When it comes to using generative AI technology on organizational networks, there are indeed several potential security concerns that organizations should be aware of. Here are some risks associated with employees using generative AI technology:
- Malicious Content Generation: Generative AI models have the capability to create realistic-looking content, including text, images and videos. However, this technology can also be misused to generate harmful or malicious content, such as deepfake videos or misleading information. If employees use generative AI technology without proper guidelines or restrictions, it could result in the dissemination of misleading or damaging content that could harm the organization's reputation or deceive others.
- Intellectual Property (IP) Protection: Generative AI models can be trained on large datasets that may include proprietary or sensitive information. If employees utilize generative AI tools on their network without adequate safeguards, there is a risk that confidential data or intellectual property might be unintentionally exposed or leaked.
- Network Vulnerabilities: Introducing generative AI technology into an organization's network may create additional attack vectors for potential cyber threats. If the AI tools or models used by employees have vulnerabilities, they could be exploited by malicious actors to gain unauthorized access to the network, inject malicious code, or perform other malicious activities.
- Data Privacy: Generative AI models often require large amounts of data for training, and organizations need to consider privacy concerns when using such models. If employees use generative AI technology that processes or stores personal or sensitive data without appropriate safeguards, it could potentially lead to privacy breaches and non-compliance with data protection regulations.
How can IT mitigate these security risks?
To mitigate these risks, organizations should consider the following measures:
- Establish clear usage policies: Develop guidelines and policies that define acceptable use of generative AI technology within the organization, including restrictions on generating harmful content or accessing sensitive data.
- Implement access controls: Restrict access to generative AI tools and resources only to authorized personnel who have undergone proper training and understand the associated risks.
- Conduct regular security audits: Continuously assess the security posture of generative AI tools and models used within the organization to identify and address potential vulnerabilities or weaknesses.
- Educate employees: Provide training and awareness programs to educate employees about the potential risks and best practices when using generative AI technology, emphasizing the importance of data privacy, IP protection and responsible content generation.
- Monitor and analyze network traffic: Implement robust monitoring systems to detect any unusual or malicious activity related to generative AI technology usage, allowing for prompt response and mitigation.
By implementing these measures, organizations can minimize the security risks associated with employees using generative AI technology on their networks and promote responsible and secure usage within the enterprise environment.
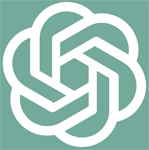
"Introducing generative AI technology into an organization's network may create additional attack vectors for potential cyber threats."
ChatGPT
Staying on the topic of security, you mentioned last time that IT can incorporate generative AI in infrastructure monitoring. How can enterprises get started today in using AI to keep their networks secure?
Using AI to enhance network security is indeed a valuable approach for enterprises. Here are some steps organizations can take to get started with AI in network security:
- Assess Security Needs: Begin by evaluating your organization's current network security infrastructure, identifying any gaps or areas where AI can provide value. Understand your organization's specific security requirements and challenges, such as threat detection, anomaly detection, or incident response.
- Identify Use Cases: Determine the specific use cases where AI can be effectively applied. For example, AI can be used for real-time threat detection, identifying patterns of suspicious network activity, analyzing logs and network traffic, or predicting potential vulnerabilities.
- Data Collection and Preparation: Gather relevant data from various sources, such as network logs, security devices and intrusion detection systems. Ensure that the data is properly collected, labeled and prepared for AI training and analysis. It's important to have diverse and representative data to train robust AI models.
- AI Model Selection or Development: Depending on the use cases, organizations can choose to either develop their own AI models or leverage existing AI solutions. There are AI-powered security platforms available that offer features like anomaly detection, behavior analysis and threat intelligence. Evaluate different options and select the one that best aligns with your organization's needs.
- Implementation and Integration: Deploy the chosen AI solution into your network security infrastructure. This may involve integrating the AI models with existing security systems, such as firewalls, intrusion detection systems or security information and event management (SIEM) platforms. Ensure compatibility and establish the necessary communication channels.
- Continuous Training and Improvement: AI models need continuous training and refinement to adapt to evolving threats and network conditions. Regularly update and retrain the models with new data to improve their accuracy and effectiveness. Stay updated with the latest advancements and research in AI for network security to incorporate new techniques and algorithms as they emerge.
- Monitoring and Alerting: Establish a robust monitoring system to analyze the outputs of the AI models in real-time. Configure alerts and notifications for detecting and responding to potential security incidents or anomalies. Combine AI-driven insights with the expertise of security professionals to validate and investigate alerts effectively.
- Collaboration and Knowledge Sharing: Foster collaboration between IT and security teams to ensure a holistic approach to network security. Encourage knowledge sharing and cross-functional understanding to maximize the benefits of AI in securing the network.
- Compliance and Privacy Considerations: Ensure that the AI implementation aligns with relevant regulatory requirements and data privacy standards. Safeguard personal and sensitive information and consider any legal or compliance obligations associated with data handling and storage.
- Regular Evaluation and Adaptation: Continuously assess the effectiveness of the AI solution in meeting security objectives. Analyze its performance, identify areas for improvement, and make necessary adjustments to enhance its efficiency and accuracy.
Remember that implementing AI in network security is an ongoing process. It requires a combination of technical expertise, collaboration across teams, and a proactive approach to stay ahead of emerging threats. By taking these steps, enterprises can leverage AI to bolster their network security defenses and improve overall cybersecurity posture.
That's a great place to stop for this installment. Next week, we'll dive into how generative AI can further be used by enterprise IT in areas outside of security.
Posted by Chris Paoli on 05/26/2023 at 11:41 AM0 comments
My name is Chris Paoli, editor at Redmondmag.com. Welcome to the first post in our new blog series Eye on Gen AI, focusing on anything and everything generative AI. What does "anything and everything" mean? Well, just like the exploding generative AI landscape, it could mean predictive language models this week and evolve to how generative AI is protecting organizational systems the next. Where it goes, we will follow and break down what it means for enterprises and IT.
In the coming weeks, we're going to focus on breaking down generative AI and what it can (and cannot) do, and what it means for enterprises. This includes shining a spotlight on hot tech like ChatGPT's predictive language modeling, discussing Microsoft's recent cadence of AI-integrated tech and investments, and looking into how IT is taking to the new tech.
For this first entry, let's break down the basics of generative AI with a conversation with said technology. We'll see what ChatGPT knows about itself, the meteoric growth of the tech and what it means for IT. Following is the lightly edited transcript of our recent chat.
Redmond: Hello ChatGPT, can you start off by breaking down what is the fundamental difference between traditional artificial intelligence and generative AI?
ChatGPT: Certainly! The fundamental difference between traditional artificial intelligence (AI) and generative AI lies in their underlying approaches and capabilities.
Traditional AI, also known as symbolic or rule-based AI, is based on explicit rules and instructions programmed by human experts. It relies on predefined algorithms and logical reasoning to process data and make decisions. Traditional AI systems are designed to solve specific tasks or problems and often require a large amount of handcrafted features and explicit instructions to perform well. These systems excel at tasks with well-defined rules and clear objectives, such as playing chess or diagnosing medical conditions.
On the other hand, generative AI, also referred to as deep learning or neural network-based AI, takes a different approach. It uses neural networks, which are large interconnected networks of artificial neurons inspired by the human brain. Generative AI models learn directly from data, rather than relying on explicit instructions. They can automatically extract relevant features and patterns from the input data, enabling them to generalize and make predictions or generate new content.
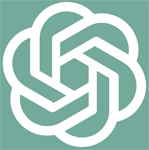
"I don't have access to specific details regarding the exact number of parameters or the size of the training dataset used for my training. OpenAI has not publicly disclosed these specifics."
ChatGPT
Generative AI models can be trained to perform a wide range of tasks, such as image recognition, natural language processing, speech synthesis and more. They are particularly powerful in tasks involving complex and unstructured data, where traditional rule-based systems may struggle. Generative AI models often require vast amounts of training data to learn effectively, and their training process involves optimizing millions or even billions of parameters.
As of May 15, how much training parameter data have you utilized?
As an AI language model, I am based on OpenAI's GPT-3.5 architecture, which was trained on a vast amount of text data. However, I don't have access to specific details regarding the exact number of parameters or the size of the training dataset used for my training. OpenAI has not publicly disclosed these specifics.
To provide some context, GPT-3, the predecessor to GPT-3.5, was trained on 570GB of text data, which included a wide range of sources such as books, articles, websites, and more. It consists of 175 billion parameters. GPT-3.5 is an extension of this architecture but with enhanced capabilities.
What industries have you seen most taking advantage of ChatGPT's features?
ChatGPT's features have found utility across various industries. Here are a few industries where ChatGPT's capabilities have been leveraged:
- Customer Support: ChatGPT has been used in customer support applications to handle inquiries, provide information, and assist customers with common questions or issues.
- Content Generation: ChatGPT has been employed in content creation, such as generating articles, blog posts, product descriptions, and social media captions.
- Virtual Assistants: ChatGPT has been integrated into virtual assistant applications, allowing users to interact with AI-powered assistants for tasks like setting reminders, answering questions, scheduling appointments and more.
- Education: ChatGPT has been utilized in educational settings to provide personalized tutoring, answer student questions, explain complex concepts and facilitate interactive learning experiences.
- Research and Development: ChatGPT has been employed in assisting researchers in exploring new ideas, generating hypotheses and conducting literature reviews. It can aid in brainstorming and knowledge discovery.
- Gaming and Entertainment: ChatGPT has been integrated into video games and entertainment applications to provide engaging and interactive virtual characters or NPCs (non-player characters) that can engage in conversation with players.
How about specifically by those in IT?
In the field of IT (Information Technology), ChatGPT's features have been leveraged in several ways. Here are a few examples of how it has been used in the IT industry:
- Technical Support and Troubleshooting: ChatGPT can assist in providing technical support to users by answering common IT-related questions, troubleshooting issues, and providing step-by-step guidance for problem resolution.
- Knowledge Base and Documentation: ChatGPT can be used to create and maintain knowledge bases and documentation repositories. It can generate explanations, tutorials, and FAQs for IT products, services, or processes, thereby enhancing the availability and accessibility of information for users and support staff.
- Code Assistance and Debugging: ChatGPT can help developers by providing suggestions, answering programming-related questions, and assisting with debugging code
- IT Project Management: ChatGPT can support project management activities in the IT industry. It can provide project status updates, answer queries about project plans or timelines, and offer assistance in managing tasks and resources.
- Infrastructure Monitoring and Alerting: ChatGPT can be integrated into monitoring systems to provide real-time insights and alerts about IT infrastructure health and performance. It can analyze system logs, detect anomalies, and provide recommendations for remediation.
- Network Security and Threat Detection: ChatGPT can assist in analyzing security logs, detecting potential threats, and providing recommendations for network security. It can help IT professionals stay updated on the latest security practices and assist in incident response.
Thanks for breaking down some of the basics for this first part of our conversion.
You're welcome! I'm glad I could provide you with these basics. If you have any more questions or if there's anything else I can assist you with, feel free to ask. I'm here to help!
Next week we will continue our conversation with ChatGPT and dive more into enterprise IT use cases, and how Microsoft is facilitating the spread of AI in its product line.
Posted by Chris Paoli on 05/18/2023 at 10:41 AM0 comments