Q&A
'What Does It Actually Excel At?' A Conversation with an AI Expert About ChatGPT
To hear one applied AI specialist explain it, the reason there are so many questions around ChatGPT is that it makes us ask questions about ourselves.
By now, you've heard of ChatGPT, the (in its own words) "conversational AI language model developed by OpenAI" that has everyone from late-night talk show hosts to the heaviest of IT heavyweights on notice. Natural language AI software isn't new, but the capabilities of ChatGPT, as well as its accessibility and ease of use, have made many of its contemporaries look primitive by comparison.
Any emerging technology has both detractors and devotees, but ChatGPT has them in spades. There are concerns about its reliability and potential for misuse, as well as fears of whole categories of workers (including creatives) being made redundant. It has its champions, too, Microsoft being the most notable. The company has committed billions to OpenAI and resolved to integrate it with its stack, starting with Azure and Microsoft Teams. This week, Microsoft upped the ante by incorporating ChatGPT into Bing and Edge, an unmistakable shot across Google's bow.
It's easy to get caught up in the wave of discourse, enthusiasm and FUD around ChatGPT, so let's temper it with an informed perspective. We caught up recently with Cameron Turner -- an applied AI expert, guest lecturer at Stanford University, a veteran of the Microsoft Office development team, and now vice president of data science at consultancy Kin + Carta -- to ask him his perspective on ChatGPT in the context of the overall IT landscape today, from its potential to its limitations. Following is our edited conversation:
Redmond: AI has been in heavy use in industry for years now. AI and machine learning, automation, robotics -- those are already ingrained in manufacturing, retail, health care. Why do you think ChatGPT has gone so mainstream so quickly, when industrial AI technologies have existed for a long time now?
Turner: ChatGPT has definitely caught the imagination of pretty much everybody. You know you've hit mainstream when late-night celebrities include it in their opening monologues. So I think we've got over some sort of tipping point with ChatGPT in terms of accessibility for everyone. And that applies both in the consumer context and in the enterprise, as well.
Part of it is -- we can unpack the term "AI" a little bit. In the last, I would say, five years, AI became this catch-all phrase that includes machine learning. So when you talk about the existing use cases that are out there making money for companies now, things like product recommendation, content recommendation, mean time to failure, estimation, demand forecasting -- all of those are really well-confined industrial problems where you can apply machine learning and specifically supervised learning. So you have training data where you have the answer, and then you train a model that sort of does the best based on its history.
To your question about why is ChatGPT pushing the boundary, it is because it can give what ostensibly seems to be fully creative answers to problems, or novel answers. In reality, what comes out of ChatGPT is combinations. It's basically a roll-up of all the history it's trained on. So then the bigger question, I think, is: What problems do we think we can take an approach where having a unique combination or an optimized set of outcomes based on that history can actually be most suitable?
And it gets into even bigger questions. What is creativity really, anyway? Did any artists ever create something completely out of thin air? Or is everything that we do -- in some ways, all of our history -- plus-plus? I think the thing that is really making people excited right now is that it seems to sort of pass the Turing test. It seems to be human, it seems to be creative. And then you have to go to that question of: Is human creativity something out of the blue sky, or is it actually a new way of contextualizing everything that we already know?
Microsoft has been heavily investing in OpenAI for a few years now, and it's been integrated into Azure, Teams and Bing. How does ChatGPT fit into Microsoft's overall trajectory since Satya Nadella became CEO?
I think there are two pieces to it. One is built into the genetics of Microsoft and opening platforms going back to the personal computer. Computers were not personal when they started, right? The PC revolution, which Microsoft was a part of, was about opening up to the masses that capability to do computing. So that, I think is very consistent.
I think Satya, though, brought in a different mindset with regard to partners and relationships with academia. That openness, that sort of glasnost toward support of Linux in the cloud, is a big step. Generally, a posture toward open source that is about expanding the state-of-the-art and supporting the entire industry based on its needs versus trying to sell a proprietary platform -- that's been very important for Microsoft's business.
Microsoft is providing a lot of leadership on this point, which I think goes back to some of its heritage. If you go all the way back to the beginning for MS-DOS, the idea was to democratize access to something that otherwise would be challenging -- going from punch cards to programming and enabling kids to write games and things like that. That accessibility point is really big, and not making access to AI be something that's a specialized skillset. I think we can fully expect that trend to continue from Microsoft.
As always, the big question for IT is security. What kind of security Pandora's box might ChatGPT potentially open in an enterprise?
We know right now that the biggest vector for ransomware and other things that plague IT today -- including keeping a balance of Bitcoin so you can pay off ransomware -- is social engineering, and social engineering at scale.
So what makes us, as a knowledge worker inside of an organization, feel like a signal is authentic versus inauthentic? It's that connection that can potentially be had. You can imagine different scenarios [of using ChatGPT] where, given all public information about all people, how could we synthesize personalized messages at scale that would then satisfy the Turing test and create that sense of authenticity? It's a huge benefit. We work on this actively in order to create personalized messaging, increasing engagement and connection between consumers and business. That's all great and people love that.
But there's a dark side to that, as well, that can be used to generate a false sense of security or authenticity. For example, your procurement department is interacting with a set of suppliers. Some third-party AI can generate invoices and start invoicing that company and it would be very hard to differentiate. So [maintaining] the foundations of security, really taking either a fully trustless system that's secure or an increased posture on security that doesn't require any social piece, is going to be really hard for organizations. We're all humans, but an email from your suppliers that you know and the one that's posing as the one you know is going to be almost impossible to tell apart.
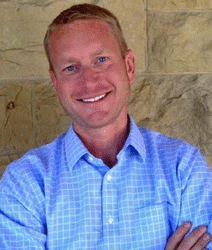
"You can't just let AI loose on an N+1 problem when it's trained off of N problems and hope that everything's going to go fine. There has to be a curation and someone there to catch the gotchas."
Cameron Turner, Vice President of Data Science, Kin + Carta
So potentially, this would be a great opportunity for an AI to be created to detect AI that's trying to pretend to be human.
Yep. Which is not a totally new thing. That's how cybersecurity effectively works. It's machine versus machine already. It's just going up another notch.
Realistically, how can enterprises determine the best use cases for ChatGPT or something like it? For instance, if you were speaking to clients of yours, how would you recommend they assess the usability of ChatGPT for their own purposes?
It's about achieving parity [with human capabilities], so in things like call centers, in order to increase human capacity by reducing workload for the most common things.
But I think the bigger question for an application like ChatGPT is: What does it actually excel at? Where does it do better than interaction with a human? One area that we're seeing some some real progress in is health care. [There was a study] looking at interactions between AI and patients versus physicians and patients, and what it found was that patients would be more honest with AI than they would be with a doctor, particularly in areas of vice, like how many drinks a day do you have.
So it's not just about how can we reduce human workload by reducing repetitive tasks that are very structured and the deterministic work that people do, and enable those people to be doing creative things or extending the state-of-the-art. But also, where is it actually better?
How should companies be telling their IT staff to prepare for technologies like ChatGPT?
I think in terms of preparation, it's really just staying in front of it. The nice thing about a lot of these technologies that we're describing is they're fully accessible. They're free and, from a trial standpoint, anyone can work and play with these things. I think that's kind of true for any technology, but especially in this space -- just get very familiar with the capability and understand what the limitations are. Just stay current. You have to be in it. It's kind of like how blockchain was five years ago. You have to play around with it to really know what it is.
I think the bigger challenges, honestly, will come from things like legal.
I was just going to ask if you think companies will have to start lawyering up if they if they intend to use ChatGPT in their business.
Either lawyer up or get ChatGPT to write legal briefs for them. [It wouldn't hurt to have] a specialist in law or IP. Because if you're generating something that's novel, is it really novel? And then how do you protect that if it's generative? It's an open question. No one has an answer to that right now.
What about standards and regulations? How should the industry go about creating a standards body for ChatGPT and these types of technologies? What is the approach there?
The challenge with taking a standards body approach to this issue is that you're operating in a context that's effectively infinite by definition. If you think about standards, it's always the thing you didn't happen to write a standard for that causes the most trouble for you. So trying to stay ahead of that is sort of a fraught task
Our approach [at Kin + Carta] is to focus on good data governance, and that governance spans both data sources and data that's used for training AI systems, but also governance on the output. Where we've landed on this question that you're asking is that human-in-the-loop systems -- where you have adjudication and curation by way of folks at organizations whose job it is to be data stewards and data governance-focused -- are the way to go. You can't just let AI loose on an N+1 problem when it's trained off of N problems and hope that everything's going to go fine. There has to be a curation and someone there to catch the gotchas, because the world will always surprise you.
ChatGPT has been the talk of the town, so to speak. Has there been a piece of FUD that you've heard or read that you would really like to correct the record on?
Computers were always meant to replace repetitive tasks. I think that what has people a little freaked out is that now it's graduating into what we thought of as being deep cognitive processes, like writing, generating music, generating poetry. No one thought that those were ever repetitive processes or something that can be fully replicated through training -- which may be a bit naive because if you study any area, by definition, you're getting up to speed on the history of that topic so that you can add to this arc.
The positive flip on that is to say, OK, maybe we are graduating as a society into another realm where we can automate a lot of things that we do up to a point [so we can] focus on purely human aspects like empathy and our judgment and our ability to synthesize new signals based on changes in the context that we operate in.